Drilling Down To Cut Readmissions
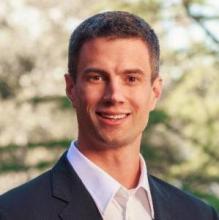
Each year millions of patients are discharged from the hospital, only to return within 30, 60, or 90 days.
Not only do patients, their families, and their caregivers suffer as a result, but hospitals, insurers, and the government waste billions of dollars that could be spent on other public health priorities. Many if not most of these readmissions could have been avoided if clinicians had effective, scalable, and timely methods for identifying not only which patients were the highest risk, but what steps should have been taken to mitigate that risk.
In recent years there has been a proliferation of readmission risk assessment models, yet readmission rates have barely budged. Fundamental flaws exist in most approaches in the areas of data, model adaptability and clinical workflow integration.
Many tools rely solely on historical patient data mined from the EHR or are disease-specific models that cannot be scaled to address all readmissions challenges. Models that rely on data collected at discharge are not timely enough to enable clinicians to take meaningful action, and ones that are not well-integrated into clinical workflow are not easily adopted.
For a readmission risk assessment tool to achieve a meaningful and long-lasting impact, these common pitfalls must be avoided at all costs.
Factors beyond our control and beyond our ability to predict may cause our fortunes to turn on a dime.
Consider the Dow Jones Industrial Average: Those who had any investments around July of 2007 remember the feelings of unabashed optimism and certainty inspired by the great bull run of the early 2000s. Unfortunately, those same investors also most assuredly remember what happened shortly thereafter, when the financial crisis of 2008 erased trillions of dollars’ worth of wealth.
A recent systematic review of readmission risk models concluded that many hospitals still model their approach to identifying high-risk patients based on historical admissions, claims data, and outdated information on patient populations.
Using these old data to model and predict readmissions is dangerous. And with increasing pressure on hospitals to reduce readmissions, this approach also runs the risk of becoming extremely costly.
Any of these folks will tell you that past performance data can not only betray you, but it may also prevent you from recognizing the obsolescence of your sources. As a result, this data may cost you a fortune.
Within the Patient Protection and Affordable Care Act, Section 3025 created a program that penalizes hospitals for excess heart attack, heart failure, and pneumonia readmissions in their Medicare populations. As a result, academic researchers and commercial enterprises built models that predicted readmissions for these diseases only.
Focusing efforts on these disease areas alone neglects other at-risk patient populations. So when the government announced new penalties for total hip and knee arthroplasty and chronic obstructive pulmonary disorder, hospitals are playing catch-up and ill-prepared to respond.
In addition, suppose that theoretically a hospital could develop risk assessment tools for the majority of their most-commonly treated diseases. Many readmitted patients have multiple chronic conditions and co-morbidities. How can clinicians determine which tools to run on which patients?
This approach would be incredibly difficult to scale and maintain.
By effectively identifying high-risk patients at the time of admission, nurses, physicians and case managers can better determine the most appropriate care plan required to best meet the individual needs of each patient.
Relying on historical utilization data or clinical factors solely to predict readmissions is ineffective and unreliable.
Case managers and physicians need a more holistic understanding of who a patient is, not the disease that they have. Using evidenced-based technology to fully evaluate patients at admission will allow clinicians to provide more focused personalized care, leading to more seamless care transitions and informed post-acute care providers.
The end goal is to improve quality of care for patients, while striving to reduce readmission rates.
Eric Heil is chief executive officer of RightCare. A version of this article first appeared at thehealthcareblog.com.